This post is inspired by many AI conversations I've had in the recent months. In particular, I'll focus on the future of creativity in a world where aspects of it become commoditised by generative AIs (Gen-AI). We will explore what creativity is, what current and future Gen-AI creativity looks like, implications for copyright, and more. This is not a post about Artificial General Intelligence. It is also not one that focuses on potential up- or downsides of AI beyond the realm of creative industry.
IT IS 2035
Let me paint a picture.
Friday morning.
O - "Hal, can you create a playlist with Ye's ‘Late Registration’ type beats but Gangsta rap like lyrics a la Tupac's ‘Makaveli’ album with a Drake voice."
H - "Excellent choice! How long should the total run time for the playlist be? Do you have a preference for the average duration of the songs?"
It's lunch time:
O -"Hal, can you give me a 45min podcast short story about Marcus Aurelius conquests in Germania. I'd love it to feature some military tactics. Narrator should be Christopher Hitchens."
H - "Happy to do so! I'll base the story on 172 BC when the Romans crossed the Danube into Marcomannic territory. Do you want the young or late Hitchens to narrate it?"
It's the evening:
O - "Hal, I'm in the mood for an early 19th century period drama with supernatural elements. Loosely base it on Christopher Nolan's ‘Prestige’. I'd like it to star Daniel Day Lewis and a young Sophia Loren. Set it in France. Give me original language with subtitles."
H - "Do you want there to be outright magic or rather seem like advanced science? Shall I make Mr Lewis speak French throughout? How long do you want the film to be?"
Saturday morning.
O - "Hal, can you create a VR space that is based on the ratification of the U.S. Constitution in Philadelphia in 1789. Emulate a discussion they may have had about the founding of the USA. I want to experience heated arguments about how to govern fairly."
H - "Exciting! Shall I also include Founding Fathers that may have not been there for the actual ratification of the Constitution? Do you want a photorealistic or stylised VR space?”
It's of course possible that the above won't be possible by 2035 (I think it'll happen sooner) but fast forward the world to whenever you think it will be feasible and let's think about the implications. Before we go there, though, let's talk about creativity.
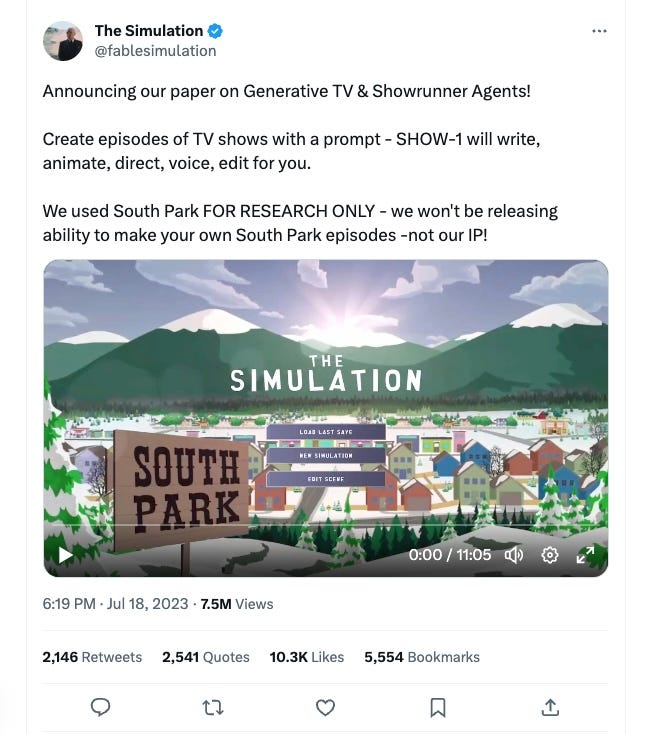
WHAT IS CREATIVITY
There is no definitive answer to what creativity is. However, the emergence of Generative AI makes exploring this question more urgent. The dictionary definition of creativity "is the ability to generate, develop, and express unique and original ideas or solutions. It involves the capacity to see connections and relationships where others do not, to take risks and challenge convention, and to make something new from what exists. It's a process that's often characterised by originality, expressiveness, and imagination."
In psychology, the creative process has been divided into several stages. One of the most common models is the four-stage model by Graham Wallas, which includes:
Preparation: This is the initial stage where one starts the process of creating. It involves understanding the problem or topic and doing research.
Incubation: This stage involves letting the problem or idea sit, often unconsciously. It's a stage of processing without direct and conscious effort.
Illumination or Insight: This is the stage where the idea or solution comes to the mind. It's often experienced as a eureka or aha moment.
Verification or Evaluation: This is the final stage where the idea or solution is tested, refined, and then finalised.
This multistep process which is required to create something new feels very antithetical to what our experience with Gen-AI is. It feels as if all the perspiration is done away with.
There are many theories about what fuels human creativity, but broadly speaking it involves divergent thinking (the generation of many unique ideas) and convergent thinking (combining those ideas into the best result). This distinction is an important one, which we will revisit later.
A 2018 study led by Roger Beaty found that creative people have increased connectivity between three brain networks: the Default Mode Network, the Salience Network, and the Executive Control Network.
Default Mode Network (DMN): The DMN includes regions of the brain that are active when a person is not focused on the outside world, typically during introspection or mind-wandering. Research suggests that the DMN is involved in creative thinking, possibly during the idea generation or incubation phase. It's thought to help us make novel connections and associations (it's also the part of the brain that most psychedelics silence but that's for another post)
Executive Network (or Control Network): This network, which includes regions like the prefrontal cortex, is involved in focusing attention and cognitive control. It may be active during the evaluation and implementation phase of the creative process when convergent thinking is required.
Salience Network: This network, including regions like the anterior insula and the anterior cingulate cortex, is believed to switch between the Default Mode and Executive Networks, depending on what's needed at the moment.
There are many different studies that explore the neurological basis of creativity. However, Beaty's is most referred to and has been validated by other experiments with fMRI scans. In other words, retreating into an inner world of ideas (rather than primarily paying attention to external stimuli), focusing on this internal stream of thoughts and evaluating the outputs of these thoughts, are crucial components of the creative process. Again, this is all together very different from what happens in Gen-AI models. There is no introspection, attention or agency in the human sense to speak of, as we will explore in the following.
CURRENT GEN-AI CREATIVITY
Let's apply our knowledge of human creativity to Gen-AI. Wallas' framework suggests 4 steps - preparation, incubation, illumination and verification.
Preparation for Gen-AI is the data set and training phase. Depending on what the particular Gen-AI is supposed to do it gets trained on a specific set of data (text, images, video, audio, etc). Most AI currently is not multimodal (it's trained on one type of input). Obviously AI can be trained on a larger data set than any human being could consume in their life time, which makes it a lot more comprehensive than humans could ever be. This makes its outputs so astounding to us.
Part of the preparation step for LLMs in particular (training visual models is different) is reinforcement learning from human feedback (RLHF). In the RLHF process, humans review and rate possible model outputs for a range of example inputs (this is a costly process). The model then generalises from this reviewer feedback to respond to a wide array of user inputs. By learning from human reviewers in this way, the model becomes more controlled, understanding, and better aligned with human values. In other words, this is the stage where we introduce purposeful bias to ensure the model doesn't generate outputs that are unsafe, nonsensical, or otherwise undesirable. So not only do we feed the LLMs human data, we also feed it human values after it's trained.
The output of all this is a vast number of weights, which represent the pattern recognition of the LLM run on terabytes of data expressed as numbers that it uses to make predictions. When a user prompts the LLM it uses these weights to predict what the next word in a sentence following the prompt could be. It arrives at millions of possible answers instantly (sort of divergent thinking) and then picks the output which has the right probabilistic outcome to be a successful answer (convergent thinking).
While we could loosely compare the weights to a "database," it's important to note that they don't represent specific facts or pieces of information. Instead, they are parameters that the model uses to make predictions based on abstract patterns. The model doesn't have access to any specific documents or sources from its training data and cannot access or retrieve information beyond what it learned during its training. In other words, the model does need any of the original data it was trained on once it has abstracted the patterns out of it. It also doesn’t actually understand any of the underlying content. It doesn't understand that London is a city or that Elon Musk is a (sometimes annoying) person. It applies its existing weights to predict sentences that are related to the prompt string of text we input. So a massive multidimensional spreadsheet with weights that autocompletes based on prompts produces outputs that seem creative. The incubation (sitting with the problem), illumination (aha moment) and verification (evaluating, testing and finalising) steps of the creative process are therefore more or less skipped by Gen-AI.
(Side bar: Chat GPT does evaluate probabilities for its outputs, which are based on a variable that we call temperature. The temperature parameter influences how these weights are used to select the next word. If the temperature is set to a high value (e.g., close to 1), the output will be more diverse and random, as the model gives relatively more consideration to lower probability options. In contrast, if the temperature is set to a low value (e.g., close to 0), the model's output will be more deterministic, as it will tend to choose the highest probability option. By adjusting the temperature, we can control the trade-off between diversity and accuracy in the model's responses. Higher temperature leads to more varied outputs but can also lead to more mistakes or nonsensical phrases, while lower temperature makes the output more focused and predictable but might also make it less creative or diverse. Chat GPT's setting is somewhere in between but can be adjusted by prompt design ("talk to me as if you are a scientist").)
Note that there is no automatic feedback loop between the user and AI. So Chat GPT doesn't get better automatically every time you use it. The corpus of prompts the user creates is available to the LLM for this particular session. This is why these models are called Feed Forward. Any potential learning that is part of interactions with users must be extracted for instance by Open AI (whenever I use Open AI in this post, you can insert Google/Meta/Microsoft instead) and then fed back into the model in the next training cycle.
(Side bar: The curious case of Sydney, where Bing Chat (based on Chat GPT) tried to convince a NYT journalist to leave his wife, prompted the Open AI team to reduce the amount of memory the LLM has in any given chat session. In other words, if you chat with Chat GPT for 2 hours, it now only retains your first prompt of the session and the last 10 or so. If it retains all the prompts it starts hallucinating. These hallucinations still happen but are mostly now limited to factual errors rather than attempts at home wrecking.)
CONVERGENT VS DIVERGENT THINKING
Remember that we differentiated two different types of thinking that are related to the creative process - divergent and convergent thinking. Divergent thinking is required to create rare original thoughts but for the most part is the act of recombining different ideas to something new. Most of human ideas are standing on the shoulders of the proverbial giants. History, first hand experiences, and the thoughts of all who influence us (even from beyond the grave) are the human training data set. We use these inputs to create divergent recombined or original thoughts.
The difference between what we do and what AI does is important. We create categories for the objects/concepts in our "database" and we deliberately remix them by understanding relations between them. The relations we create between the objects/concepts are not statistical but they are based on their inherent qualities (as viewed by meat bag humans). This seems very different from running an autocomplete algorithm based on weights and probabilities in a database. AI does pattern recognition better than any human ever could, but it doesn't know what pattern it's actually uncovering (it is baffling that it still works so well). It may be true that the human creative process is not as mysterious as it currently seems, but it is definitively more valuable than what is happening in current Gen-AI models. In particular, original thought seems to be in a completely different category. Einstein coming up with general relativity and understanding that space-time is a continuum was a genuinely original thought. Newton creating differential calculus from the ground up was genuinely original thought. Our unknown ancestor creating the wheel was an original thought. These products of original thoughts transcended the prior human training data set. The current Gen-AI design doesn't seem to allow for this.
Once divergent thinking has created a host of options, convergent thinking hones in by evaluating them and fleshing out the most promising contender. Gen-AI emulates this process really well. It has run pattern recognition on the sum total of a lot of humanity's knowledge represented in weights, which allows it to generate millions of options to choose from (divergent "thinking") when presented a prompt. By evaluating probabilities it autocompletes (convergent "thinking") the text that is deemed amazingly creative by humans (once again without understanding the content in the same way a human would). It may be that the size of the data set and therefore the infinite possibilities for recombination of text make it so compelling.
The fact that we have invented something truly creative that operates in this way suggests several things about creativity:
Pattern recognition: A significant part of creativity involves recognising and manipulating patterns, something that LLMs excel at. They can help us understand how much of what we perceive as "creativity" is based on rearranging existing ideas in new ways.
Influence of diverse inputs: LLMs are trained on diverse data, which allows them to generate diverse outputs. This echoes the creative principle that exposure to a wide range of inputs, experiences, and perspectives can foster creativity.
Value of convergent and divergent thinking: LLMs can generate many options (like divergent thinking) and then hone in on a single output based on their training (like convergent thinking), reflecting the balance of these two types of thinking in the creative process.
Remember, an LLM can't learn from new experiences, reflect on its thoughts, or change its thought processes based on feedback, which are all critical aspects of human divergent thinking. It can't generate ideas that are truly novel or that go beyond the patterns in its training data. While an LLM can generate diverse outputs that might resemble divergent thought, it's not capable of the originality, insight, or adaptability that characterise true divergent thinking in humans.
So let's revisit the definition of creativity. Gen-AI does "generate, develop and express unique ideas" but it doesn't do so by seeing "connections and relationships where others do not". It doesn't "take risks to challenge convention". It does create something "new from what exists". It likely does this so well because it holds orders of magnitude more connections between data than a human brain ever could. Despite it not understanding any of the data it does a great job at creating undeinably creative outputs.
COPYRIGHT?!
Now let's imagine Gen-AI gets better at generating all different media types - text, images, audio, video, 3d objects - feels very likely. Let's further assume it becomes multi-modal so that it can output all different types of media - i.e. audio plus video from text. This is actively being worked on and will happen. With enough progress cycles this gets us to my 2035 scenario - a world in which a prompt leads to "high quality" entertainment.
You may believe that the outputs by Gen-AI could never be good enough to compete with what humans are churning out. I would argue that most of the content, which is consumed by humans is quite generic. Many top 100 games, books, songs and blockbusters would support this thesis. I love Marvel movies for what they are but some of their scripts are so extremely weak that a random plot generator would mostly do a better job. We also have enough of a sense by looking at Chat GPT-4 outputs to realise it does a decent job already comparatively. So what happens when the majority of what we currently call "creative" output becomes commoditised by Gen-AI?
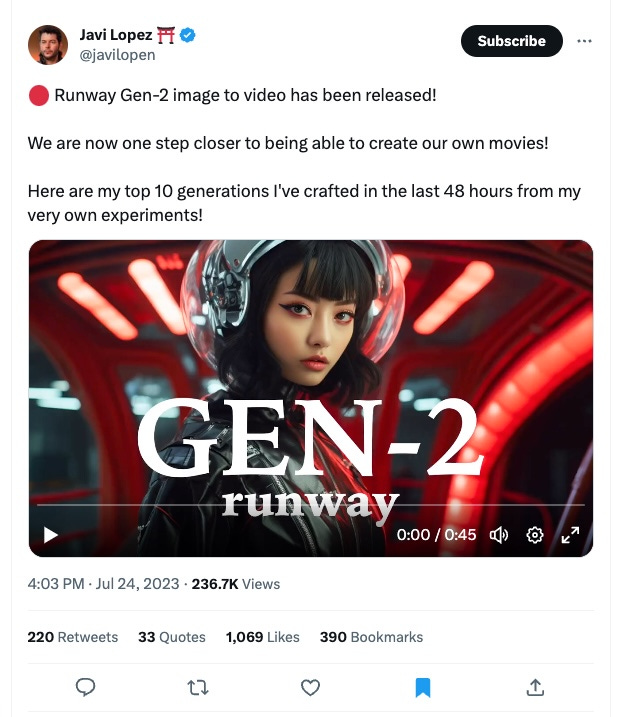
To answer that question let's address the elephant in the room - who owns the training data? Without the open nature of the internet there would be no Gen-AI. It is the terabytes of data which can conveniently be scraped off sites and databases that acts as the training data set. But when people and cooperations uploaded this data to the web, they didn't realise that they are de facto enabling Gen-AIs to eventually replace them. So what does the law say about this? I will focus on US law for now but the situation doesn't look clear anywhere. Training generative AI models on copyrighted data sets is complex and not universally defined. Here some of the considerations:
Fair Use: One of the primary defense against copyright infringement is the concept of "fair use," which allows limited use of copyrighted material without permission for purposes such as criticism, parody, news reporting, research, and education (so it is OK for Chris Rock to make fun of Star Wars). Whether the use of copyrighted data for training an AI model constitutes "fair use" would depend on several factors, including the purpose and character of the use, the nature of the copyrighted work, the amount and substantiality of the portion used, and the effect of the use on the market for the original work. The latter seems to be the most important point - what if the effect of the use destroys the market for the original work?
Transformative Use: A key aspect of "fair use" is whether the use is "transformative," meaning it adds something new to the original work or uses it for a different purpose. If an AI model is trained on copyrighted material and produces new, original output, some might argue that it's a transformative use. However, this area of law is still being developed and interpreted, and courts may not universally agree on this point. It will likely require a much better understanding of how these Gen-AI models work (which currently not even its creators possess) to figure out if what's happening is transformative technically.
Contractual Agreements: If the data is obtained under a license or terms of service, those terms may explicitly allow or prohibit certain uses of the data, including for machine learning. This seems like a straight forward one to settle - if there is no ML clause in your content licensing agreement with iStockphoto, you shouldn't be training a model on their assets.
Privacy Laws: Depending on the nature of the data, there could also be privacy laws or other regulations that apply, such as the General Data Protection Regulation (GDPR) in Europe. This may be relevant when it comes to creating digital likeness that is based on humans without their explicit opt-in.
Of course, I don't know how this will play out. It’s impossible to comprehensively address all the implications for all different stakeholders here (what happens to journalists, news, bloggers, authors, musicians, script writers, etc). I'm sure there are plenty of lobbyists currently trying their darnest to wine and dine legislators to carve up the future.
Creators and rights owners are fighting back. Getty Images is suing Stable Diffusion (text-to-image Gen-AI) as they trained their model on Getty's copyrighted material. Stable Diffusion argues this is fine under fair use. In Hollywood writers and actors are striking to ensure their contracts don't contain AI clauses. Actors being replaced by their likeness and writers training Gen-AI that will replace them in the future are real threats to their livelihoods. Sarah Silvermann is suing OpenAI and Meta as she believes the companies used her copyrighted comedy writing to train their models. Apparently they acquired her works from “shadow library” websites like Bibliotik, Library Genesis, Z-Library, and others, noting the books are “available in bulk via torrent systems.” Your pictures could also be part of a training data set without you knowing. After searching for my name "Omid" on HaveIBeenTrained. I found images of me in training sets (you can request to be removed).
Irrespective of everything that is currently going on, how should the future of all this play out? If we revisit my opening examples there are a few questions that pop up. What happens when we use someone's likeness (check out this ad)? What if we prompt a piece of content that is explicitly based on something else (or its likeness)? What happens with out of copyright content or the likeness of deceased people? These are all complicated questions that depend on the legal, digital platform and process architecture we have created but what if we could disregard reality and create an imaginary model?
UTOPIA...
An ideal model would allow any creator to fingerprint content. AI training and models then would have to keep track of such fingerprints (they currently don't even know how content is aggregated into those ominous weights). Every time an AI then uses my fingerprinted content in any context, I accrue royalties (similar to how spins work in the music industry). If we were able to design such a system, creating useful content that in turn AI uses to produce content would reward me financially. This is much better than a future where I sign away “my likeness” whenever I publish anything (text, image, my face) and never get paid again. This way AI becomes a distribution channel for my remixed ideas on top the traffic my content would otherwise get. In many ways, this is how music already operates and ensures that people don't use popular copyrighted songs in Youtube videos without paying the piper.
There are of course issues with this. I may be creating content by remixing other people's stuff. How should we deal with that? In a recent court case Ed Sheeran was cleared from allegedly violating copyright of Marvin Gaye's song Let's Get it On. The likeness of the songs is undeniable but they are ultimately different enough for Sheeran to make money on content, which is clearly inspired by Marvin Gaye. It seems that we don't mind copying by other humans as long as it is leading to output that seems different enough. All of Zara's clothing is ultimately a rip off of runway designs. Inspiration is accepted in many industries.
What happens if I use AI to create content, which then makes it back into AI training sets? It becomes a slippery slope that's hard to account for. A just model would involve storing all the input contributions on a ledger and pay out a revenue share based on contribution no matter how many times things get remixed (songwriting works like this to an extent). This seems hard to do technically, but we will have to find some sort of solution, as otherwise the 2nd remix of Scarlett Johansen's likeness (which may look more attractive) might displace her all together (pfff who am I kidding). Of course, this system will have to deal with IP trolls who may end up creating many versions of stories inspired by the Iliad/Bible/Koran/Bhagavad Gita to get paid a royalty whenever this out of copyright popular content is used. Let alone solving the problem of verifying whether a piece of content is really yours to fingerprint.
I know at this point I've likely discredited my own proposition to an extent, but these are not simple issues and there don't seem to be any proactive suggestions other than luddite law suits. One rare and interesting proactive approach comes from Grimes. She has taken on this problem head on and published Elf tech. This tool allows anyone to use their own singing voice input to create a song with Grimes voice. If Grimes decides to publish the song, she will share revenue with the users. Should the user decide to publish the song, they will have to share (a bigger amount) of the proceeds with Grimes.
There are plenty of startups that are working on AI that is ultimately built on a private training set. These will allow for people to create their own (or company's) likeness, which can be prompted. For only 2 bucks you could prompt Omid LLM to create a blog posts for you! A fitting analogy here is that Open-AI (et al) has showed up to a knife fight with plasma weapons from the future, stolen everyone's content and trained an alien AI. These new startups are trying to level the playing field by handing out plasma weapons to the rest of us. If Getty Images and Omid can create their own models, we can monetise our content/likeness rather than Open-AI (et al). What that potentially means in the future are a lot of content silos behind paywalls. If there is no attribution or royalty sharing model allowing for some fair value exchange, the public internet becomes a place to be mugged at scale with plasma weapons by content devouring AI companies.
(Side bar: There are projects like https://site.spawning.ai/spawning-ai-txt which allow you to create a text file stored in your website's root directory to signal to AI scrapers that you don't want to be part of their shenanigans. This is inspired by robot.txt, which signalled to search engines to not index a specific site. While this seems like a good idea, it doesn't seem to be universally adopted or respected and comes after Open AI (et al) has already created a GPT 4 with 1.76 trillion parameters.)
(Side bar: Most of the architecture for startups to build their own LLMs and Gen-AI models is open. Google to their credit made a breakthrough with transformers, which form the basis for a lot of the LLM breakthroughs and open sourced this. Open-AI has since been against open sourcing (ironic given their name), as it believes this technology is too powerful to be given to the "average Joe". Meta has taken the opposite stance (maybe to stay relevant) and has just recently published its second model Llama 2 to the public (including training and weights info, which it didn't publish previously). It is fascinating to see these different philosophies clashing. There is a lot of debate going on as to what the right approach is to this technology but that is beyond the scope of this post. However, it is undeniable that AI startups are very happy about Meta's moves as it reduces their reliance on OpenAI.)
FUTURE GEN-AI CREATIVITY
There will be a few world changing breakthroughs even before we get Artificial General Intelligence. While the current approach to scaling Gen-AI is increasing parameters (i.e. the amount of data we feed them during training), the future might look drastically different.
Imagine a world where AI companies don't need Terabytes of data to to create a competent Gen-AI. This is already the case for narrow applications. As mentioned some startups allow companies and people to create their personal AI models. Illustrators can train a model that creates images that bear the likeness of the input material with only 10 images as input. There are examples for this for email writing, contract drafting, image creation, etc. Of course, these models are very narrow and won't be able to write a Shakespearean poem about Tinder competently. But what if Open-AI (et al) finds a way to train Chat-GPT 42 with 10,000 pieces of content? Royalties and attribution would be out of the window.
You may think it is impossible to capture the full complexity of the human language and creativity in 10,000 pieces of content (I wonder how much content is in my active memory that allows me to write this blog post right now). I think it could come worse actually. While the domain of Chess and Go are combinatorially simpler than the realm of language, Deepmind's AlphaGo and AlphaGo Zero provide us with a cautious tale. AlphaGo was trained by being fed a dataset of roughly 30 million moves played by humans. It reliably won against human opponents. Deepmind then decided to come up with a new approach. AlphaGo Zero was trained with no human input as data set. It was given the rules of the game and was told to play itself. In its first 9 hours of existence it played 44 million games against itself. After 2 hours it was good enough to win against every human all the time.
There is something really exciting and terrifying about this. AlphaGo Zero took an approach to playing Chess and Go that was not encumbered by past human bias, which was part of its predecessors training data, thereby creating moves that no human had ever played or considered to play. It has mastered truly divergent thinking capable of creating original thoughts. It would sacrifice its Queen, because it knew that 7 moves later this would allow it to check mate. Humans have trouble thinking like that. Exploring the possibility space without priors is probably required to solve a lot of the problems in science. However, not being able to feel the pain for the loss of a Queen might also lead to unacceptable sacrifices in the pursuit of unravelling the mysteries of this cosmos.
Another component that we should not ignore while we dream up future scenarios are potential illegitimate uses of copyright, which are already prevalent on the web. While law abiding websites may not misuse the likeness of someone to create content, the open source availability of AI tech spawns a cottage industry of pages that allow you to create whatever you want. Most recently an unknown user published a decent but fake Drake and The Weekend song. Platforms like Spotify and Youtube eventually took it down but only after millions of views had triggered the respective labels to trigger rights violations on the respective platforms.
It is possible that there will be a return to using torrents and pirate bay sites en masse to exchange Gen-AI created content, which isn't copyright cleared. If you think current deepfakes are impressive, you haven't seen anything yet. Sites like deepswap.ai allow users to generate faceswap videos, photos, and GIFs in a few clicks. While deepswap might want to be above board, there are plenty of sites that don't care. The nightmare scenario of ending up in a believably looking deepfake porn is already real. Once you tour the seedy side of the AI world, there is plenty to find. It starts with “relatively tame” Gen-AI prompted porn images and ends with feeding an AI Instagram pictures for it to automagically remove the clothes of whoever is in the picture (no I didn’t try it out).
Fast forward all this to 2035 and you will realise that any attempts to realise my Utopia suggestion above might be ill fated. All hope is not lost. We have somehow managed to give up our bad habits of downloading music and movies because the UX of streaming sites is just so damn convenient. Hopefully, the illegitimate side of the internet will stay small because it will be cumbersome. However, the world will undeniably become more unsettling for anyone who is happy to put their likeness on the web.
CONCLUSION
This is not meant to be a doomer post. Gen-AI will allow us to explore the possibility space of imagination and creativity in unprecedented ways. The conversation about AI has to have a healthy component that highlights the continued empowerment of humans through this technology. The question is what we will lose in the process. Right now AI is remixing existing content on the web. Should the original creators of said content get fair compensation? Yes. This seems like a problem we can fix. Original creations will still be the domain of humans (for now). The question is how much is originality really valued. Looking at top 100 of any content type, not very much. Do some people care for originality? Yes. Those same people will continue to care.
In the mean time, we need to figure out what to do with the not so original content creator's incomes. Maybe the way to make money in the future is to design great prompts. Maybe some of my prompts above will create content that less imaginative prompt designers or lazy users would like to consume. The previously creative class might be now empowered to create an explosion of content that is not bound by production budgets and times. But how can we make sure the prompt designers get paid? I won't bet on regulators. One can hope that the market will create a platform with revenue share for prompt designers, where the underlying content and likeness is fairly compensated. One can imagine that this site would attract the most valuable content and most creative prompt designers versus places that don’t compensate either.
I for one am excited for the richness of experience that these tools can bring us. As someone that considers themselves creative but not particularly talented, I am excited that AI will allow me to produce things that were previously out of my reach. I am concerned by the collateral damage that the adoption of such technologies always brings. "Legacy" businesses like Netflix might disappear in favour of a Gen-AI prompt content creation services. Many people may lose their jobs, however some might get new more fun jobs. Some very few companies may rip off all of humanity’s creativity to create dominant Gen-AIs that don't fairly compensate the original creators. We should try our hardest to avoid these negative outcomes. I naively hope that consumers and the market will reward the right behaviour, if we create the right narratives now. This is me attempting to do my part.
What happens to creativity in a world when AI can create original thoughts unbound by a human training data set? Nobody knows, but let's take this one post at a time.